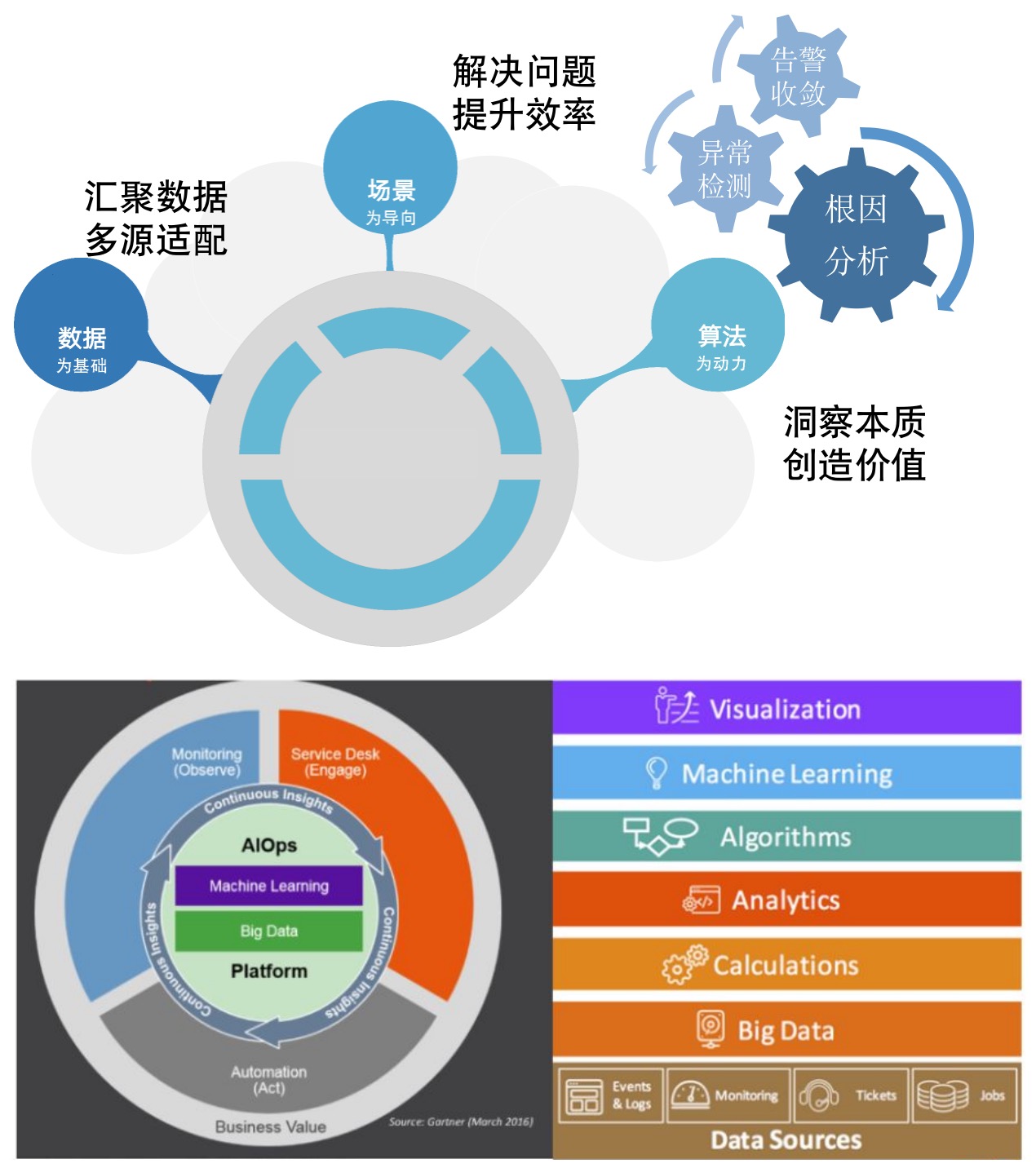
Blue Elephant-Operation and Maintenance Operation Data Zhongtai (HCS Edition)
The artificial intelligence of the IT operation (AIOPS) platform combines big data, AI machine learning and other technologies, and supports all major IT operation functions through active, personalized and dynamic insights. Major banks in the financial field, GA and power grids in various parts of the country, as well as large industrial enterprises, etc., their information network structure and related application systems are very large. In daily work
- Knowledge map, an ontology model of abstract operation and maintenance objects on the basis of CMDB, establish resource maps, and form dynamic resource portraits
- Trend prediction, diminishing dimensions through the analysis of the main component, classification and marking through the automatic classifier, and then obtaining the indicator trend prediction model
- The container load predicts that more accurate load prediction is performed using the load changes between the container, and the load data modeling is the load of the next moment.
- Dynamic baseline, timing data enters the real -time digital warehouse, and there is a management model for data sources and indicators in digital warehouses. According to the constructed indicator model
- The network traffic abnormal detection, the network map is constructed, the links at all levels are integrated, the sequential data is merged, and the network alignment framework is deeply confronted
Major banks in the financial field, GA and power grids in various parts of the country, as well as large industrial enterprises, etc., their information network architecture and related application systems are very large. In daily work, there will be related faults such as the network and business. If the operation and maintenance method of the positioning and positioning of manual analysis is difficult, it is difficult to adapt to the increasingly growing business needs. The expanding IT system, the increasingly complex system architecture, and the massive IT operation and maintenance data are as good as the operation and maintenance personnel who use the traditional DevOps. In 2017, Gartner, the world's largest authoritative IT research and consultant consulting company, put forward the concept of AIOPS in its own report. Gartner's definition of AIOPS is: artificial intelligence of the IT operation (AIOPS) platform combines big data, AI machine learning and other technologies, and supports all major IT operation functions through active, personalized and dynamic insights. After that, the entire operation and maintenance industry quickly took AIOPS to the inevitable direction of solving operation and maintenance problems in the future. Therefore, based on the deep experience of IT operation and maintenance operations of the Public Prosecution Law for more than 10 years, our company has developed its own AI -enabled data in the blue elephant.
Based on the three -dimensional real -time monitoring, full coverage of resource management, and multi -themed data warehouse as the base, the data, algorithms and models are applied to the tasks and processes of automated operation and maintenance operations, the frontier of the benchmark innovation, competition and productivity -big data, sea, sea, sea Lianqi launched the data of the data -blue elephant.
In the data, the public component layer in the data is systematically connected and data collection through Flume .. These mainstream tools are set up, and Party A is built through various data pre -processing tools such as Kylin. Provide offline, real -time, and map computing capabilities. Support data modeling and knowledge map generation, and provide a series of algorithm libraries. At the level of service support, the construction of data sets for operation and maintenance and operations, continuously expanding abnormal detection, trend prediction, and academic library of root cause analysis to better support intelligent data services.
Application performance monitoring products By actively monitoring the real experience of the end user and the operating status of the application system, the end -to -end performance monitoring and analysis of the business system can help enterprises actively discover and handle the abnormal phenomenon of all links of the business system, ensure that the application system can meet the expectations Service level. Application performance monitoring and the core links of the business application system correspond to one by one, including mobile, browser, application and host modules, and realize the mobile APP user experience management management, web page user experience management, back -end application system performance monitoring analysis, host performance monitoring Essence
Data collection through an open public interface adaptation platform, including unified authentication, data access, and API packaging. In this framework, you can flexibly access any third -party monitoring data, as well as third -party application and network monitoring data. After access, due to the heterogeneity of the access data of each three -party platform, a flexible data mapping provides a flexible data mapping Tools are stored in the Data CMDB, indicator library, and alarm library with uniform standards.
First of all, we provide a flexible data access framework that can be used to take the data of the third -party monitoring system to the third -party monitoring system, thereby building the data warehouse of Party A, providing offline computing, real -time computing and graph computing power, supporting support, supporting support Data modeling and knowledge diagram are generated, and a AI empowerment engine is constructed, dedicated to the construction of models such as abnormal detection, root cause analysis, capacity planning, service portrait in operation, and providing intelligent data services.
The data warehouse uses a snowflake model. Based on the various data of the ODS layer, various types of data models are constructed in the DWD layer. The theme library supports the large -screen display of various themes.
The knowledge map first abstracts the ontology model of the operation and maintenance object on the basis of CMDB. 2. Establish a resource map based on the ontology model, reflect the relationship between resource association, and introduce the time -sequential knowledge map of time -sequential data such as indicators to form a dynamic resource portrait.
The trend predicts that for the historical indicators in the digital warehouse, first use the main component analysis and other means to reduce the dimension, extract features, classify and marked by automatic classifiers, and build training data sets. Then perform model training to obtain the indicator trend prediction model, and provide the accuracy of the output through weight optimization. For example, we found that the container business types are different, computing containers, access containers, and their loads are different. By automatic classifiers distinguish different types of containers, predict the load, and adjust the containers that are not obvious through weighted. The benefits of prediction are first to understand the trend of change, and have forward -looking in operation. At the same time, it can assist in discovery and warning.
The container load predicts that in the online business system deployed by microservices, the relevant business is deployed to different containers, and a business flow will cause the load index of the upstream and downstream container to change the load index in synchronization. Therefore, we consider using the load changes between container to perform more accurate load prediction. The load data is modeling as a figure. The container is used as the vertex in the figure. The influence of dynamic deployment and expansion capacity. The correlation between this container is dynamic. We cannot obtain the topology relationship between nodes in advance. Therefore, we have built a related learning model to generate a variable -time sequential graph G, which is based on spectrum based on spectrum. The diagram constructs a load prediction model, and the G to the G to the spectrum domain is expressed, the law of learning sequences; then converts from the spectrum domain to the frequency domain to learn the rules of the sequence; finally The load.
Dynamic baseline, timing data enters the real -time warehouse, and there is a management model for data sources and indicators in the digital warehouse. According to the constructed indicator model, the historical and real -time timing data is prepared to distinguish between stable, cyclical, and fluctuations. Type, then select the appropriate abnormal detection method for different features, detect the abnormal points, and write the abnormal library from the abnormal feedback. The fluctuation data is difficult to detect abnormal detection by the year -on -year, month -on -month and fixed threshold method, and a deep learning model must be tested.
Regarding equipment abnormal detection, we have implemented an abnormal detection method based on single indicators. First use the method of interval volatility to extract features, and then integrate spatial characteristics and timing characteristics through convolutional neural networks and long -term memory, and enrich the characteristics of data. Finally, the classification judgment module is detected abnormal, and the classification judgment module adopts a full connection for non-linear relationship extraction. The K-σ principle is judged and the state of the device is detected.
在网络流量异常检测方面,我们首先构建了网络图谱,整合各级链路,融合时序数据;进而设计了深度对抗网络对齐框架,该框架首先使用深度对抗学习来发现复杂的网络映射,以对齐两Embedding distribution of individual networks. Then, use the mapping function (generator) we learn to perform an effective nearest neighbor node alignment, so as to effectively detect the abnormal network traffic.
In terms of service abnormal testing, we adopt the idea of predicting first and then testing to solve this problem. The service call diagram of the time -sequential topology and the log text diagram containing the log semantic relationship is made to make features to obtain a service prediction model, so as to predict the service calls that are most likely to occur in the next time. At the same time, we have trained a unsupervised abnormal detection model through historical indicators to discover the service index abnormality. During real -time testing, we predict the next service that may be called through the service prediction model. Targeted detection according to the predicted service can reduce most of the useless log detection, more effective discovery abnormalities and alarm.
All users
Commodity pricing
Commodity price = number of order interval 1*range specification unit price 1+order range number 2*range specification unit price 2.
Example: The call rate is 0.3 yuan/minute for a part of no more than 3 minutes, and the part of more than 3 minutes is pressed at 0.2 yuan/minute; if the user calls for 8 minutes, the fee is charged at 3*0.3+5*0.2 = 1.9 yuan.
Commodity price = order of order*number of sections.
Example: The call rate is 0.3 yuan/minute for no more than 3 minutes, and if it exceeds 3 minutes, it is 0.2 yuan/minute; if the user calls for 2 minutes, the fee is charged at 2*0.3 = 0.6 yuan; if the user calls for 8 minutes, it will be collected. The cost is 8*0.2 = 1.6 yuan.
Commodity price = order quantity*unit price.
The price of the product is the price of the page.
Platform support scope After -sales service time: 5 * 8 hours After -sales service content: cooperative consultation, product use and implementation service Service hotline: (010) 5638 6788 Service mailbox: market@mt-hirisun.com Contact address: Room 701, Building 7, Building, No. 5, Fengchuang Science and Technology Park, No. 18, Science and Technology Development Zone, Beijing Economic and Technological Development Zone, Beijing
Click to view "Open source software statement"
Order this product is to agree with you "Commodity service protocol"